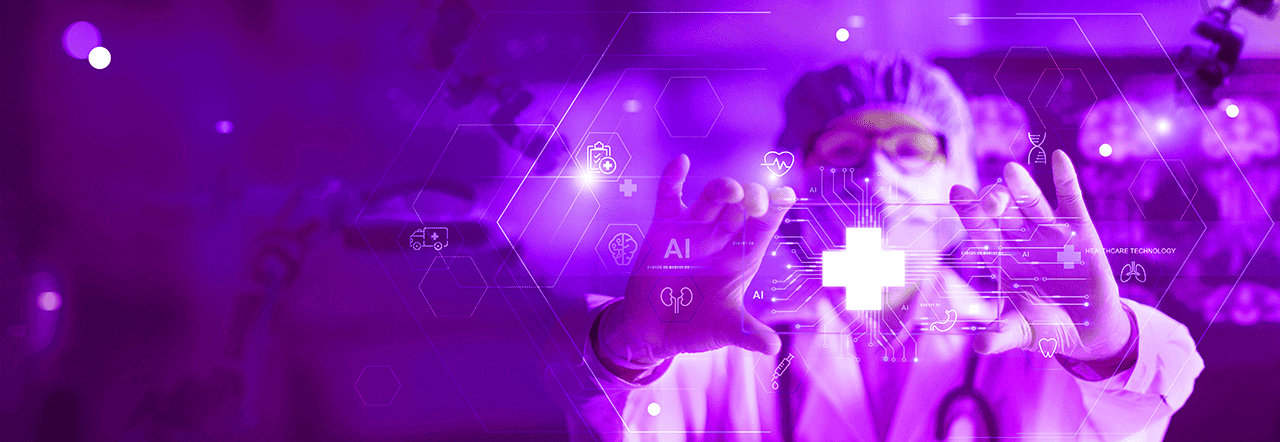
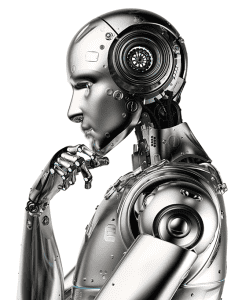
Artificial intelligence (AI) is only as good as the data it’s trained on. Putting it bluntly – Rubbish in, Rubbish out.
To ensure more accurate, immersive and engaging AI experiences, AI engines must be trained on large volumes of high-quality data. But preparing the AI data you need to train your machine learning (ML) model is a monumental task that can consume up to 80% of AI project time, leaving little time to focus on developing, deploying and evaluating your AI applications. One possible solution? Working with the right AI data partner to deliver the exact AI training data you need.
But how much does AI data cost?
AI data vendors take different approaches to pricing AI training data. Some vendors price hourly based on actual time spent preparing the data, some price based on the number of data points delivered, while others price based on productivity considering the time it takes to complete each data task and the total number of data tasks required.
Regardless of pricing approach, the cost of AI data ultimately depends on four key components: thats the 4 P`s
- People
- Productivity
- Process
- Place
People
When budgeting for AI training data, the people you want to collect, annotate/label or validate your AI data can have a significant impact on the cost of your data. Below are the people factors that impact AI training data pricing:
- Number of participants – what is the total number of participants you want to collect, annotate, or validate data?
- Geographic, demographic, sociographic, or physiologic requirements – do you need participants to have specific features e.g. come from certain countries or regions, belong to a particular age range or ethnicity, speck specific languages or dialects with certain accents, have a particular skin tone etc.?
- Specialized skills – does your data task require a resource to have specialized knowledge e.g. multilingual skills, computer programming skills, legal expertise, medical doctors, specific hobbies, etc. or can anyone perform the task?
Productivity
Another key component that must be considered when budgeting for your AI training data is task productivity. The following task productivity factors will affect your AI data budget:
- Data type – what type of data e.g. text, audio/speech, image, or video, has to be collected, annotated or validated?
- Number of data points – how many data points do you require from each participant? A data point is one output from one participant such as one image, one video clip, one audio utterance, etc.
- Time to complete a data point – how long would it take a participant to perform one data point from start to finish excluding setup and training time?
Process
The process used to perform your AI data collection, data annotation and data validation tasks also influences the cost of AI training data. The following procedural factors play an important role in AI data pricing:
- Training – do the data collection, annotation, or validation tasks require project-specific training prior to completion?
- Reasoning – do the tasks require reasoning where the answer is not explicitly mentioned in the data type but the pieces of information can be used to deduce an answer.
Place
The setting in which data tasks must be performed can also greatly impact AI data pricing. Below are a few criteria related to place that must be considered:
- On-site vs. remote – do you require resources to be on-site or can they perform tasks remotely? If on-site, does the project need to be performed in a certified facility?
- Environment – does a specific environment/scenario have to be set up?
- Equipment – is specific equipment such as cameras, recording devices, or props that a typical individual is not likely to have access to required?